Sushko I, Applicability Domain of QSAR models. Excerpt
Given a predictive model and an additional set of new experimental measurements (referred to as “library”), it is possible to correct the model by taking into account these measurements. The process is called LIBRARY model correction (because we complement a model with a “library” of experimental measurements) and is performed as follows.
To obtain a corrected prediction for a molecule J, we calculate the original (noncorrected) prediction
given by the original model and find K molecules from the “library” {Ji, i = 1..K} nearest to the molecule being predicted. The nearest compounds are defined by the correlation coefficient in space of model predictions.
Then, we calculate the expected residual for the molecule J as the average residual for the K nearest compounds according to the following expression:
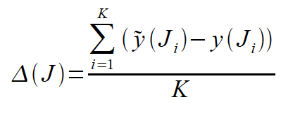
Finally, we correct the original prediction by subtracting the average residual:

Thus, the LIBRARY correction assumes that for a new compound a model will behave similarly to compounds from the “library”. Such technique is especially useful in case if retraining of the original model is infeasible due to high computational complexity or unavailability of the original training set. The LIBRARY technique was introduced by Tetko and was shown to significantly increase the prediction accuracy for the lipophilicity and distribution coefficient models.
http://mediatum.ub.tum.de/node?id=1004002